Data Quality: a monster with many heads
Erik Schaap sep 21, 2021
This interview was done to contribute to the masterclass Data Quality and Data Governance performed for Outvie.
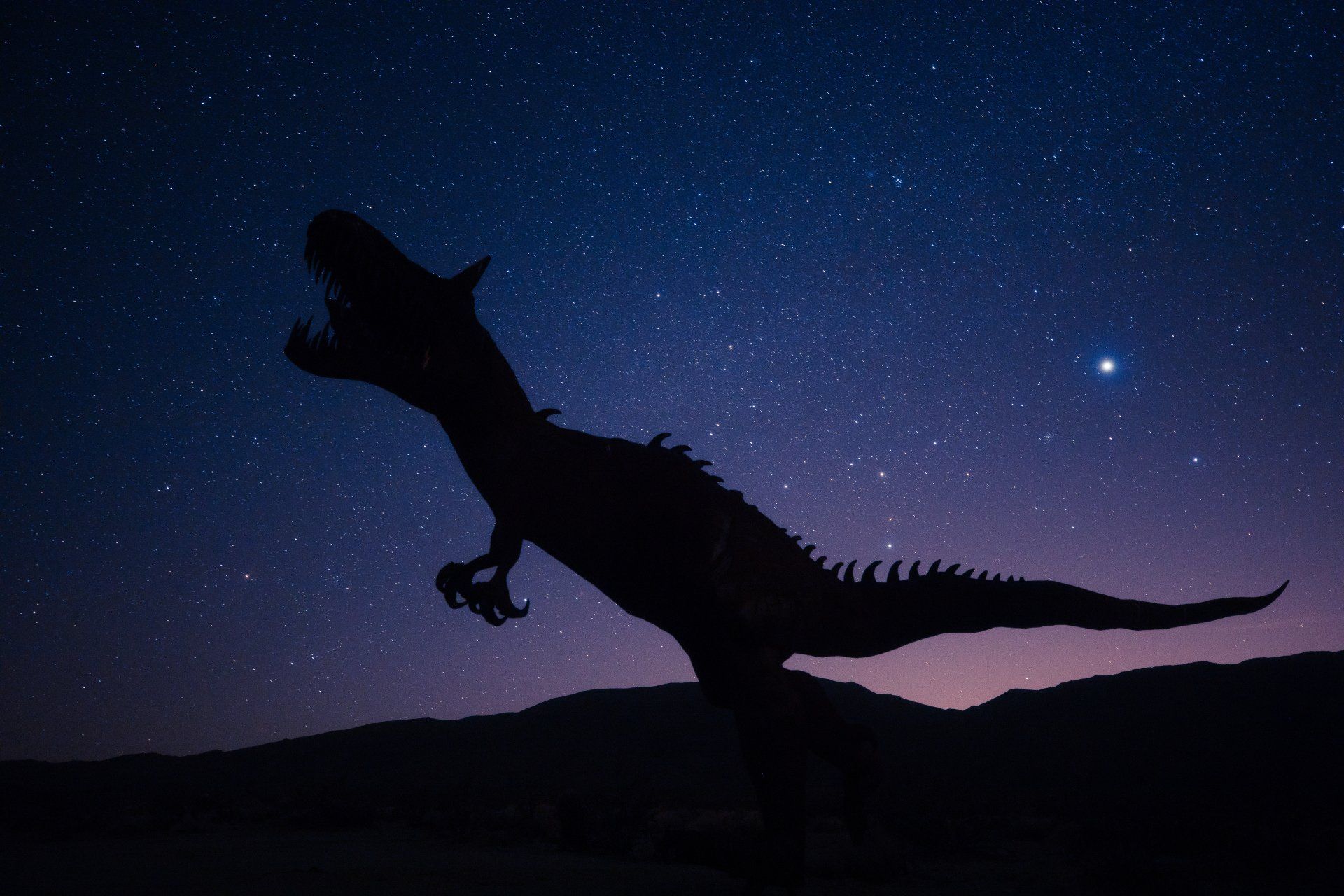
Good data quality touches on the essence of an organization. The consequences of using incorrect, late or incomplete data can be disastrous. Certainly for organizations that want to become data-driven or want to use technologies such as AI and algorithms for their business processes. We asked Erik Schaap, Data Management and Analytics specialist and senior lecturer of the masterclass Data Quality and Data Governance, about the current issues and pitfalls surrounding the assurance and continuous improvement of data quality in organizations.
Why is it important to be actively involved with data quality?
Organizations that endorse the strategic importance of information will have to pay structural attention to the quality of that data. After all, if the data does not meet expectations, negative consequences such as extra costs, loss of trust in organizations or even fines can be the result. The quality of data can be disrupted in many places when processing data. That is precisely why we recommend a framework to keep our finger on the pulse and to be informed in time in the event of disruptions in the quality of data.
And how is it possible that data quality is often not high on the administrative agenda?
In general, administrators assume working systems ('otherwise we cannot be successful for many years anyway') and the responsibility for the correct processing of information lies with the IT department. However, the data quality problem is a monster with many heads. Finding the causes of problems is no easy task. The knowledge of data quality and the urgency of data quality often falls short in management, as a result of which less attention is paid to it.
What are challenges in measuring data quality dimensions?
The biggest challenge in assessing data quality is defining it using data quality dimensions. Think of dimensions such as completeness, uniqueness, accuracy, consistency, etc. The business determines the requirements that are set for these dimensions. By entering into discussions with the business, a solid foundation can be laid under the data quality program. In this way it can be determined which requirements the data must meet. Moreover, the environment of an organization is changeable, which means that the requirements that the organization places on data also change. New legislation, for example in the field of privacy, creates new business rules that have an impact on a large number of systems.
Where is data quality usually invested in an organization and under which administrative role does data quality actually belong?
Because good data quality touches on the essence of an organisation, it is of great importance that senior management takes responsibility for the data quality policy. Because data quality is in fact a business issue, we would like to see this task assigned to the CFO or CEO. In more and more organizations a Chief Information Officer or Chief Data Officer is taking a seat on the board with data quality in the portfolio.
What type of organization and culture is needed to guarantee data quality?
Ensuring data quality in an organization is very specific. Depending on the type of organization and the way in which decision-making already takes place, a choice can be made for a central, decentralized or hybrid model. To do this, first analyze the current organizational structure. How independently do the departments or regions operate? Are there major differences in information needs and goals? How does the decision-making process and the implementation of decisions proceed?
What are pitfalls in continuously improving data quality?
To continuously improve data quality, you will have to implement a data quality strategy that should be anchored in the company culture over time. This is a lengthy process with many pitfalls, the most common of which are: not feeling or no longer feeling urgency, too few short-term successes and insufficient communication about the changes.
How can developments such as AI, Machine Learning, Data Science contribute to the quality of data?
Measuring and improving data quality is generally done with specialized, automated tools. The vendors of these tools are increasingly implementing artificial intelligence (A.I.) and machine learning techniques to discover trends in the data. In general, the knowledge of data science becomes easier to apply in organizations because the modern tools are more accessible (citizen data scientists). Algorithms can predict expected values of measurements and compare them with the actual measurements. The occurring deviations from the prediction model can then be assessed by data stewards. This provides new insights into the data and the possible cause